Mandatory Provident Fund (MPF) is a compulsory saving scheme for the retirement of residents in Hong Kong. Over the past 25 years, the MPF has succeeded in encouraging household participation in securities markets and played a crucial role in improving financial inclusion in Hong Kong. However, it has faced ongoing criticisms for its low annualized rate of return. With the upcoming launch of the e-MPF platform to integrate disparate savings schemes into a single digital system, it presents a well-timed opportunity to drastically improve Hong Kong’s primary retirement savings system.
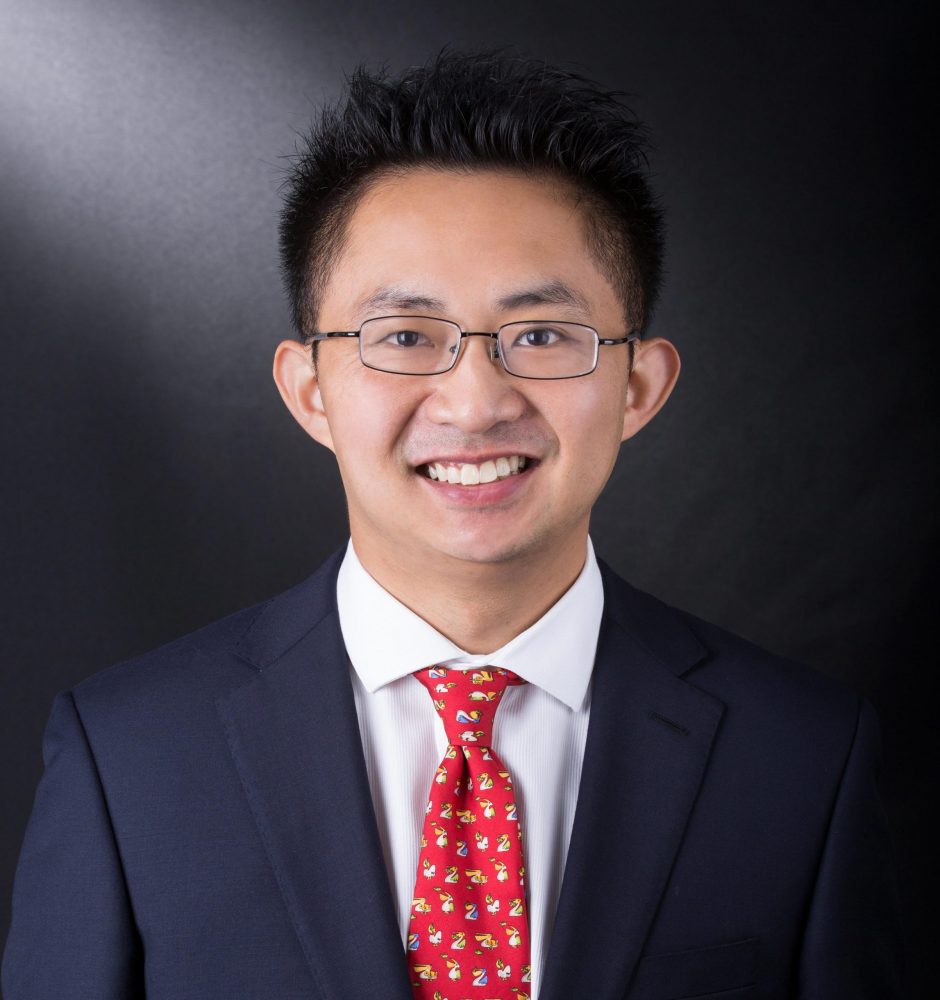
2859 1049
KK 923
- PhD, Cornell University
- BA, Dartmouth College
Dr. Alan P. Kwan is an academic currently serving as an Associate Professor of Finance at the University of Hong Kong. He also serves as program director for the Master’s of Finance in Financial Technology.
Dr. Kwan’s interest in finance was influenced by years working in the financial industry, including roles at a major global macro hedge fund and a quantitative trading firm specializing in energy markets. His research focuses on two main themes: regulatory economics, and the economics of intangible capital. In regulatory economics, he aims to understand the implications of regulatory events and laws on financial market participants. His work on intangible capital aims to understand how a firm’s knowledge, technology, and information acquisition create value. To study these, he uses big data and machine learning techniques in collaboration with corporate partners with vast datasets.
He has published a variety of papers in outlets including American Economic Review, Management Science, Science Advances, Journal of Financial Economics, and the Journal of Financial Quantitative Analysis. He has also presented his research at top selective conferences in his field, including the Western Finance Association, American Finance Association and National Bureau of Economic Research, winning several best prize awards.
- Corporate finance
- Innovation
- Investments
- Financial advice
- “Does Regulatory Jurisdiction Affect the Quality of Investment-Adviser Regulation?” with Ben Charoenwong and Tarik Umar, American Economic Review, 109(10), 2019, 3681-3712.
- “Social Connections with COVID-19-affected Areas Increase Compliance with Mobility Restrictions” with Ben Charoenwong and Vesa Pursiainen, Science Advances, Nov 2020.
- “Crowd-judging on Two-Sided Platforms: An Analysis of In-group Bias” with Alex Yang and Angela Zhang, Management Science, 2023.
- “Stress Testing Banks’ Digital Capabilities: Evidence from the Covid-19 Pandemic” with Chen Lin, Mingzhu Tai and Vesa Pursiainen, Journal of Financial and Quantitative Analysis, 2023.
- “Capital Budgeting, Uncertainty and Misallocation” with Ben Charoenwong, Yosuke Kimura, and Eugene Tan, Journal of Financial Economics, Vol 53, 2024, 103779.
- “Regtech: Technology-Driven Compliance and its Effects on Profitability, Operations, and Market Structure” with Ben Charoenwong, Zachary Kowaleski and Andrew Sutherland, Journal of Financial Economics, Vol 154, 2024, 103792.
- “Bargaining power in the market for intellectual property: Evidence from licensing contract terms” with Gaurav Kankanhalli, Journal of Empirical Legal Studies, 21(1), 2024, 109-173.
- “The Paradox of Innovation Non-Disclosure: Evidence from Licensing Contracts” with Gaurav Kankanhalli and Kenneth Merkley, American Economic Journal: Applied Economics, 16(4), 2024, 220-256
Innovative firms must trade off disclosing to investors and maintaining secrecy from competitors. We study this trade-off in a sample of IP licenses mandatorily disclosed by US public firms, whose contents can be temporarily redacted. Hand classifying the redacted information, we find that firms with valuable IP in competitive markets redact IP information more often. Markets react positively to the redaction of IP information, consistent with theoretical predictions rationalizing a separating equilibrium in which nondisclosure signals more valuable IP. Our results suggest that credible nondisclosure partially resolves information frictions for innovative public firms when facilitated by sophisticated investors.
Compliance-driven investments in technology—or “RegTech”—are growing rapidly. To understand the effects on the financial sector, we study firms’ responses to new internal control requirements. Affected firms make significant investments in ERP and hardware. These expenditures then enable complementary investments that are leveraged for noncompliance purposes, leading to modest savings from avoided customer complaints and misconduct. IT budgets rise and profits fall, especially at small firms, and acquisition activity and market concentration increase. Our results illustrate how regulation can directly and indirectly affect technology adoption, which in turn affects noncompliance functions and market structure.
Disputes over transactions on two-sided platforms are common and usually arbitrated through platforms’ customer service departments or third-party service providers. This paper studies crowd-judging, a novel crowdsourcing mechanism whereby users (buyers and sellers) volunteer as jurors to decide disputes arising from the platform. Using a rich data set from the dispute resolution center at Taobao, a leading Chinese e-commerce platform, we aim to understand this innovation and propose and analyze potential operational improvements with a focus on in-group bias (buyer jurors favor the buyer, likewise for sellers). Platform users, especially sellers, share the perception that in-group bias is prevalent and systematically sways case outcomes as the majority of users on such platforms are buyers, undermining the legitimacy of crowd-judging. Our empirical findings suggest that such concern is not completely unfounded: on average, a seller juror is approximately 10% likelier (than a buyer juror) to vote for a seller. Such bias is aggravated among cases that are decided by a thin margin and when jurors perceive that their in-group’s interests are threatened. However, the bias diminishes as jurors gain experience: a user’s bias reduces by nearly 95% as experience grows from zero to the sample median level. Incorporating these findings and juror participation dynamics in a simulation study, the paper delivers three managerial insights. First, under the existing voting policy, in-group bias influences the outcomes of no more than 2% of cases. Second, simply increasing crowd size through either a larger case panel or aggressively recruiting new jurors may not be efficient in reducing the adverse effect of in-group bias. Finally, policies that allocate cases dynamically could simultaneously mitigate the impact of in-group bias and nurture a more sustainable juror pool.
In canonical models of investment dynamics under uncertainty, “time-to-build” in investment decisions implies that uncertainty negatively impacts firm values and aggregate capital productivity. However, capital budgeting, which involves ex-ante information acquisition and state-contingent investment decisions, can potentially ameliorate time-to-build frictions. Reduced-form evidence using firm-level data on sales and investment expectations and errors supports both mechanisms. Incorporating capital budgeting into a standard investment model, our calibrated model reveals that state-contingent investment planning and information acquisition reduce aggregate productivity losses by 41% and 17%, respectively. Moreover, gains from planning accrue primarily to less productive firms, while information acquisition benefits higher productivity ones.
Due to the aforementioned socioeconomic issues faced by the city, among other reasons, hundreds of thousands of Hong Kong’s working-age population and their families have emigrated abroad. Simultaneously, hundreds of thousands of people, some attracted by the Hong Kong government’s ambitious talent programs, have arrived in Hong Kong in the past year. What are the implications of these population flows on the city’s labor force and talent pool? Dr. Alan Kwan, Prof. Heiwai Tang, and Dr. Michael B. Wong have analyzed LinkedIn profile data and government statistics to evaluate Hong Kong’s labor market and economic prospects.
The Dodd-Frank Act shifted regulatory jurisdiction over “midsize” investment advisers from the SEC to state-securities regulators. Client complaints against midsize advisers increased relative to those continuing under SEC oversight by 30 to 40 percent of the unconditional probability. Complaints increasingly cited fiduciary violations and rose more where state regulators had fewer resources. Advisers responding more to weaker oversight had past complaints, were located farther from regulators, faced less competition, had more conflicts of interest, and served primarily less-sophisticated clients. Our results inform optimal regulatory design in markets with informational asymmetries and search frictions.