Does a "free lunch" really exist? Some online shopping platforms let consumers disclose if they received products for free or at a discount when writing reviews. Although these reviews for free products are not the majority, they can energize online communities where reviews are limited. The lack of objectivity in free product reviews can trigger self-regulation within online communities, prompting more users to provide fair feedback. Voluntary disclosure policies can effectively boost the number of reviews at a lower cost than hiring experts to write positive reviews.
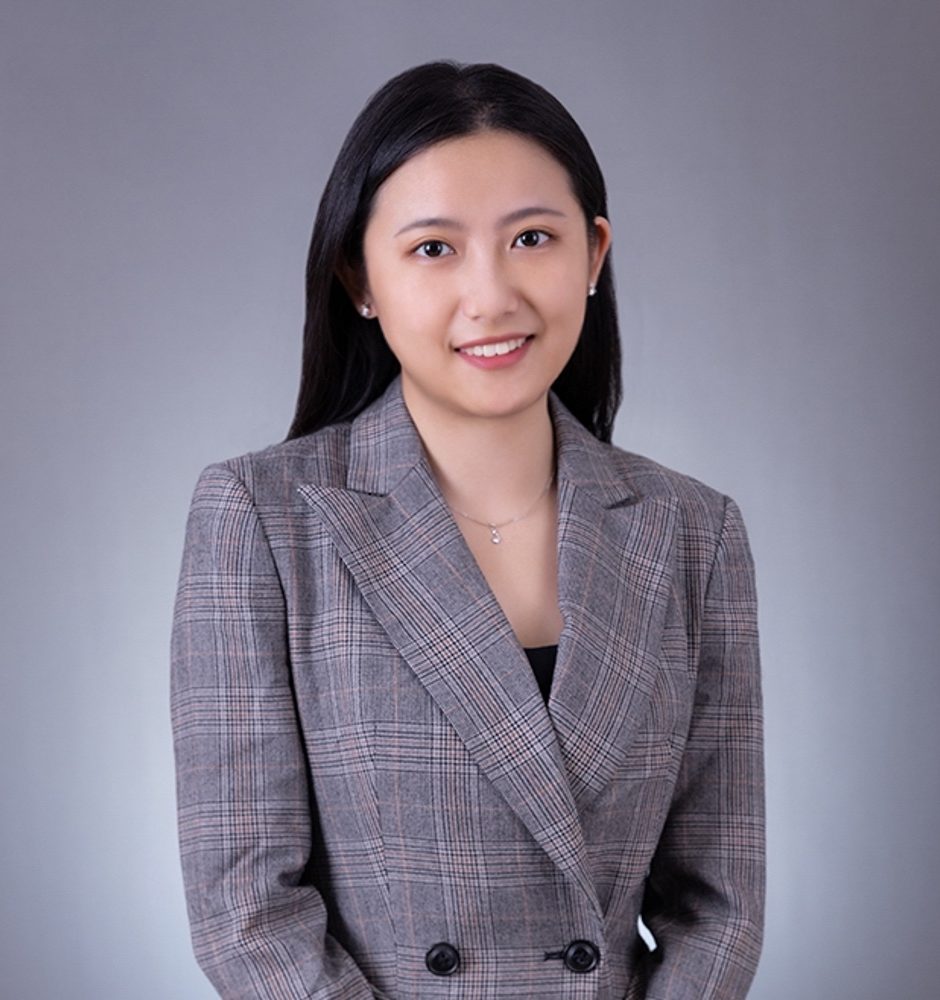
3917 1672
KK 1308
Does a "free lunch" really exist? Some online shopping platforms let consumers disclose if they received products for free or at a discount when writing reviews. Although these reviews for free products are not the majority, they can energize online communities where reviews are limited. The lack of objectivity in free product reviews can trigger self-regulation within online communities, prompting more users to provide fair feedback. Voluntary disclosure policies can effectively boost the number of reviews at a lower cost than hiring experts to write positive reviews.
We collaborated with a leading fast-moving consumer goods (FMCG) manufacturer to investigate how intelligent image processing (IIP)-based shelf monitoring aids manufacturers’ shelf management by using data from a quasi-experiment and a field experiment. We discovered that such artificial intelligence (AI) assistance significantly and consistently improves product sales. Several underlying mechanisms were revealed by our quantitative and qualitative analysis. First, retailers are more likely to comply due to the greater monitoring effectiveness enabled by AI assistance. Second, the positive effect of IIP-based shelf monitoring partially persists after it is terminated, implying that human learning takes place. Third, the value of IIP-based shelf monitoring can be attributed to independent retailers rather than chain retailers. Since the degree of contract heterogeneity is the major difference between these retailers in terms of monitoring, this finding further suggests that AI is relatively more scalable when coping with more heterogeneous instances. Apart from these great benefits, we demonstrate the low marginal costs of implementing IIP-powered shelf monitoring, which indicates its long-term applicability and potential to generate incremental value. Our research contributes to several literature streams and provides managerial insights for practitioners who consider AI-assisted operational models.
We investigate an editorial review program for which a review platform supplements user reviews with editorial ones written by professional writers. Specifically, we examine whether and how editorial reviews influence subsequent user reviews (reviews written by noneditor reviewers). A quasiexperiment conducted on a leading review platform in Asia, based on several econometric and natural language processing techniques, yields empirical evidence of an overall positive effect of editorial reviews on subsequent user reviews from the platform’s perspective. First, more reviews are provided for restaurants that receive editorial reviews. In addition, these reviews discuss substantive topics while also including a discussion on other topics, leading to a net increase in content length and variety. They also are more neutral in sentiment and are associated with lower rating valences. Further analysis of the mechanism reveals that the subsequent user reviews of the restaurants that receive editorial reviews become more similar to the editorial reviews in regard to topics, sentiment/rating, length, and readability, indicating a herding effect in how to write a review as the main driver of the change in the subsequent reviews. We further empirically isolate this herding effect among long-time reviewers. The findings suggest that review platforms could use an editorial review program not only to boost the quantitative aspect of user reviews but also, to manage the qualitative aspect as well.