This article addresses the challenges in classifying textual data obtained from open online platforms, which are vulnerable to distortion. Most existing classification methods minimize the overall classification error and may yield an undesirably large Type I error (relevant textual messages are classified as irrelevant), particularly when available data exhibit an asymmetry between relevant and irrelevant information. Data distortion exacerbates this situation and often leads to fallacious prediction. To deal with inestimable data distortion, we propose the use of the Neyman–Pearson (NP) classification paradigm, which minimizes Type II error under a user-specified Type I error constraint. Theoretically, we show that the NP oracle is unaffected by data distortion when the class conditional distributions remain the same. Empirically, we study a case of classifying posts about worker strikes obtained from a leading Chinese microblogging platform, which are frequently prone to extensive, unpredictable and inestimable censorship. We demonstrate that, even though the training and test data are susceptible to different distortion and therefore potentially follow different distributions, our proposed NP methods control the Type I error on test data at the targeted level. The methods and implementation pipeline proposed in our case study are applicable to many other problems involving data distortion. Supplementary materials for this article, including a standardized description of the materials available for reproducing the work, are available as an online supplement.
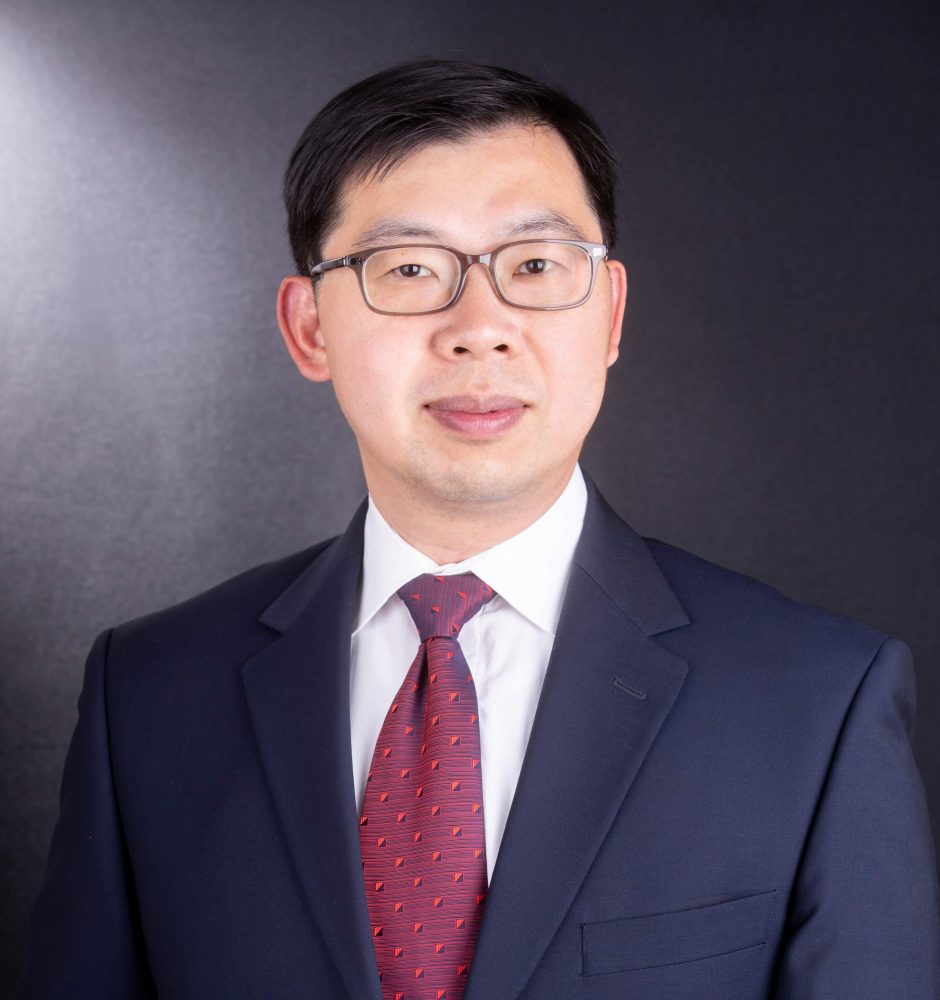
Prof. Yanhui WU
Economics
Management and Strategy
Associate Professor
3917 8508
KK 931
Publications
1Mar
1 Mar 2021
Journal of the American Statistical Association