Volatility forecasting is crucial to risk management and portfolio construction. One particular challenge of assessing volatility forecasts is how to construct a robust proxy for the unknown true volatility. In this work, we show that the empirical loss comparison between two volatility predictors hinges on the deviation of the volatility proxy from the true volatility. We then establish non-asymptotic deviation bounds for three robust volatility proxies, two of which are based on clipped data, and the third of which is based on exponentially weighted Huber loss minimization. In particular, in order for the Huber approach to adapt to non-stationary financial returns, we propose to solve a tuning-free weighted Huber loss minimization problem to jointly estimate the volatility and the optimal robustification parameter at each time point. We then inflate this robustification parameter and use it to update the volatility proxy to achieve optimal balance between the bias and variance of the global empirical loss. We also extend this Huber method to construct volatility predictors. Finally, we exploit the proposed robust volatility proxy to compare different volatility predictors on the Bitcoin market data and calibrated synthetic data. It turns out that when the sample size is limited, applying the robust volatility proxy gives more consistent and stable evaluation of volatility forecasts.
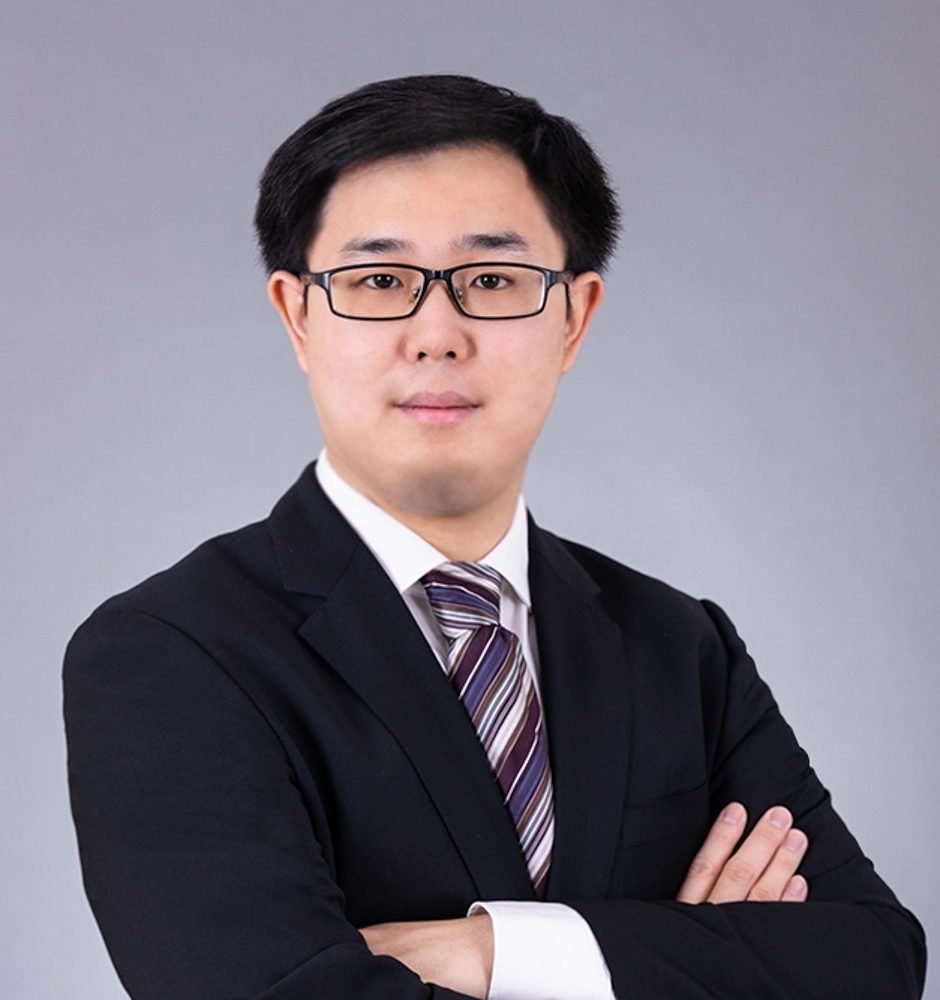
3917 1617
KK 1319
- PhD in Operations Research and Financial Engineering, Princeton University, 2016
- BS in Mathematics and Physics, Tsinghua University, 2011
Dr. Weichen Wang joined HKU in 2021 as an Assistant Professor. He obtained his PhD in Operations Research and Financial Engineering from Princeton University in 2016. After graduation, he joined Two Sigma Investments as a quantitative researcher where he worked on applying machine learning for equity market forecasting. Dr. Wang also served as a Visiting Lecturer at Princeton University for Spring 2020. Before his PhD, he received his bachelor’s degree in Mathematics and Physics from Tsinghua University in 2011.
Dr. Wang’s research areas include big data analysis, econometrics, statistics and machine learning, and he is particularly interested in the factor structure of the financial market and real-world applications of machine learning and deep learning. His works have been published in top journals including Annals of Statistics, Journal of Machine Learning Research, Journal of Econometrics etc.
- Business Statistics
- Research Methodologies in Business Analytics
- Quantitative Trading / AI in Finance
- Big data analysis
- Machine learning
- Econometrics and asset pricing
- Factor model and low-rank structure
- Semi-parametric and robust statistics
- Fan, J., Lou, Z., Wang, W., & Yu, M. (2024). Ranking Inferences Based on the Top Choice of Multiway Comparisons. Journal of the American Statistical Association, 0(0), 1-14.
- Wang, W., An, R., & Zhu, Z. (2024). Volatility Prediction Comparison via Robust Volatility Proxies: An Empirical Deviation Perspective. Journal of Econometrics, 239(2), 105633.
- Wang, W., Han, J., Yang Z., & Wang Z. (2021). Global Convergence of Policy Gradient for Linear-Quadratic Mean-Field Control/Game in Continuous Time. International Conference on Machine Learning (ICML), 10772-10782.
- Fan, J., Wang, W., & Zhu, Z. (2021). A Shrinkage Principle for Heavy-Tailed Data: High-Dimensional Robust Low-Rank Matrix Recovery. Annals of Statistics, 49(3), 1239-1266.
- Fan, J., Wang, W., & Zhong, Y. (2018). An l∞ Eigenvector Perturbation Bound and Its Application to Robust Covariance Estimation. Journal of Machine Learning Research, 18(207), 1–42.
- Fan, J., Liu, H., & Wang, W. (2018). Large Covariance Estimation through Elliptical Factor Models. Annals of Statistics, 46(4), 1383–1414.
- Wang, W., & Fan, J. (2017).Asymptotics of Empirical Eigen-Structure for High Dimensional Spiked Covariance. Annals of Statistics, 45(3), 1342–1374.
- Fan, J., Liao, Y., & Wang, W. (2016). Projected Principal Component Analysis in Factor Models. Annals of Statistics, 44(1), 219–254.
The coronavirus pandemic has interrupted the lives of many, but a lucky few is bestowed with the serendipity to rethink on their life choice. Taking this opportunity to soul search, Dr. Weichen Wang has decided to follow his heart and restarted his academic journey. Joining us in July 2021, Dr. Wang is as an Assistant Professor in Innovation and Information Management.