The ease of customer data collection has enabled the widespread personalization of content and services in digital platforms. We examine personalization in a hitherto unaddressed context: that of mobile app distribution. Specifically, we develop a comprehensive framework for the personalized ranking of app impressions, leveraging revealed preferences embedded in consumer clickstream data. To improve platform revenues, the framework jointly accounts for consumer utility and cost per action (CPA) margin, which is the revenue earned by the platform per app installation. To this end, we specify a structural model of click and installation choices, jointly estimated as a function of a comprehensive set of numerical (screen rank, quality, and popularity) and textual (titles, descriptions, and reviews) covariates. Our novel data set is at the granular user-impression level and uniquely includes app CPA margins paid to the platform. We conduct a series of policy experiments to quantify the value of personalization. Specifically, we show that a personalized hybrid margin and utility margin ranking scheme outperforms other personalized methods, including those based on utilities alone or a combination of utilities and margins. Overall, our analysis demonstrates how platforms can leverage routine consumer clickstream data to personalize the ranking of app impressions, thereby more effectively monetizing mobile app distribution.
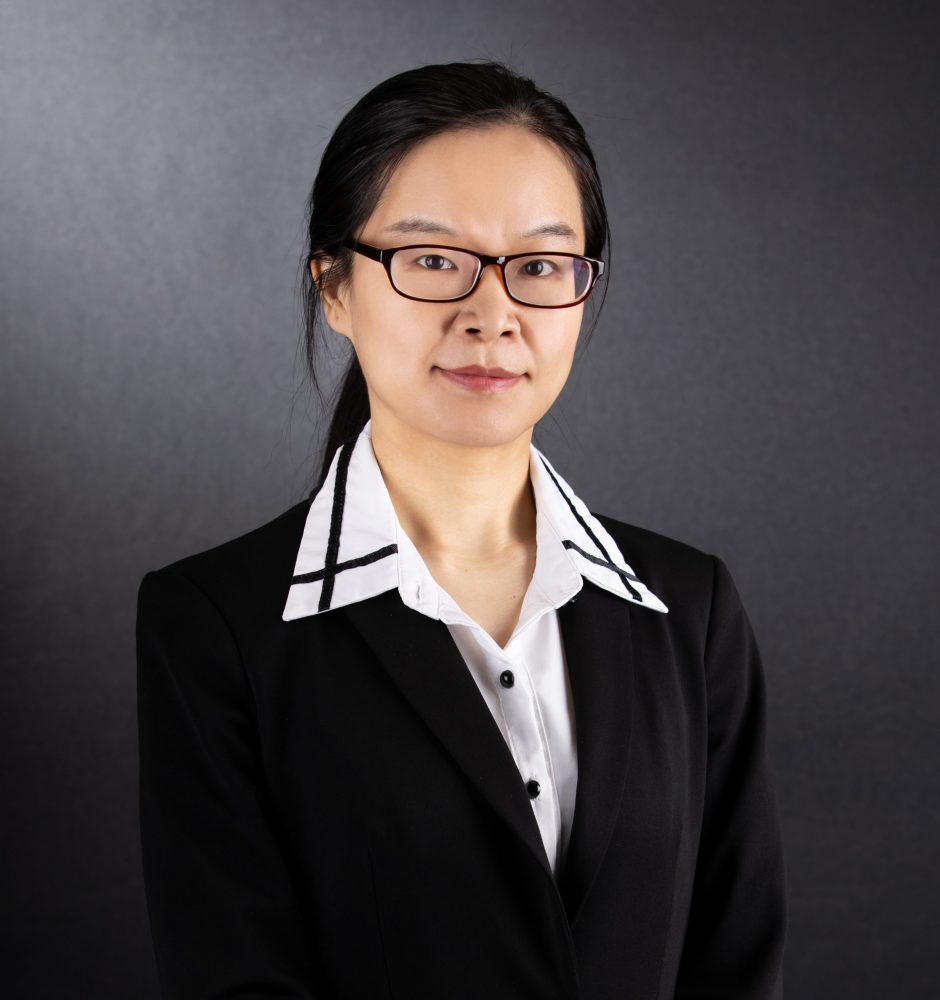
Prof. Shengjun MAO
Innovation and Information Management
Assistant Professor
3917 1637
KK 813
Academic & Professional Qualification
- Ph.D., University of California, Irvine
- M.A., Peking University
- B.S., Beijing University of Chemical Technology
Biography
Shengjun Mao joined the University of Hong Kong in 2020. Her research mainly focuses on practical problems in mobile app economy and digital platforms including ranking strategies, monetization strategies, content curation, recommenders and usage habits etc. Her research is largely grounded on multi-methods including econometric analysis, field experiments, and theoretical modeling, along with machine learning, and natural language processing techniques. |
Research Interest
- Mobile App Economy
- Recommender Systems
- Digital Marketing
- Gamification
Selected Publications
- Mao, S., Dewan, S., and Ho, Y. “Personalized Ranking at a Mobile App Distribution Platform.” Information Systems Research, forthcoming.
Recent Publications
1Sep
1 Sep 2023
Information Systems Research