In flexible manufacturing lines with delayed differentiation, the production process may fluctuate sharply when a control action is performed. As a result, the steady-state analysis algorithm is inaccurate for these production lines, and transient behavior studies have become crucial. However, dynamic analysis remains unexplored compared with the well-established theoretical system of steady-state analysis. Therefore, in this study, we propose a fast algorithm for predicting the production process performance in the delayed differentiation-based flexible production line under operation control. We first formulate practical problems existing in the auto, food, and furniture industries into a mathematical formation. Then, we offer closed-form formulae for predicting the production process performance using the built stochastic model in the production line with three machines. We also propose an algorithm to predict the performance of a production line having more than three machines. The proposed methods were verified to be highly accurate through comparison experiments. In terms of theoretical contributions, this study offers a research foundation for other transient-based studies. From a practical perspective, the proposed algorithms can be employed to predict the production process performance of processing lines under production control in advance.
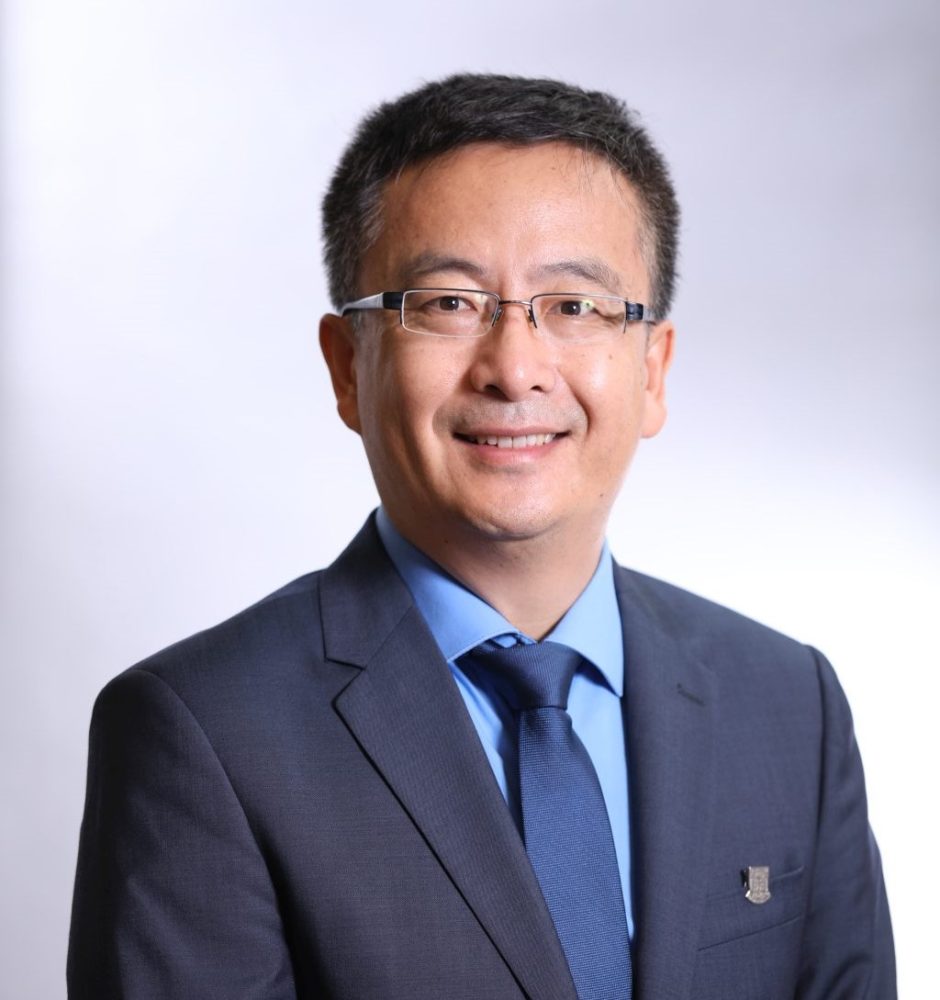
KB 1040
Professor Shen obtained his PhD from Northwestern University, USA in 2000. He started his academic career as Assistant Professor at the University of Florida in the same year, and joined the University of California, Berkeley in 2004, where he rose through the academic ranks to become Chancellor’s Professor and Chair of the Department of Industrial Engineering and Operations Research and Professor of the Department of Civil and Environmental Engineering. Professor Shen joined HKU in 2021. Internationally recognized as a top scholar in his field, Professor Shen is a Fellow of the Institute for Operations Research and the Management Sciences (INFORMS), a Fellow of the Production and Operations Management Society (POMS), a Fellow of the Hong Kong Academy of Engineering Sciences, and a former President of POMS.
- Integrated Supply Chain Design and Management
- Data Driven Logistics and Supply Chain Optimization
- Design and Analysis of Optimization Algorithms
- Energy Systems Optimization
- Transportation System Planning
- Fundamentals of Supply Chain Theory, co-authored with Larry Snyder, was published by Wiley in August, 2011.
- Integrated Modeling for Location Analysis, co-authored with Ho-Yin Mak, Foundations and Trends in Technology, Information and Operations Management. Download the book
- Handbook of Supply Chain Analysis in the E-Business Era (Springer)
Problem definition: Content promotion policies are crucial for online content platforms to improve content consumption and user engagement. However, traditional promotion policies generally neglect the diffusion effect within a crowd of users. In this paper, we study the candidate generation and promotion optimization (CGPO) problem for an online content platform, emphasizing the incorporation of the diffusion effect. Methodology/results: We propose a diffusion model that incorporates platform promotion decisions to characterize the adoption process of online content. Based on this diffusion model, we formulate the CGPO problem as a mixed-integer program with nonconvex and nonlinear constraints, which is proved to be NP-hard. Additionally, we investigate methods for estimating the diffusion model parameters using available online platform data and introduce novel double ordinary least squares (D-OLS) estimators. We prove the submodularity of the objective function for the CGPO problem, which enables us to find an efficient (1−1/?) -approximation greedy solution. Furthermore, we demonstrate that the D-OLS estimators are consistent and have smaller asymptotic variances than traditional ordinary least squares estimators. By utilizing real data from a large-scale video-sharing platform, we show that our diffusion model effectively characterizes the adoption process of online content. Compared with the policy implemented on the platform, our proposed promotion policy increases total adoptions by 49.90%. Managerial implications: Our research highlights the essential role of diffusion in online content and provides actionable insights for online content platforms to optimize their content promotion policies by leveraging our diffusion model.
This work provides performance guarantees for solving data-driven contextual newsvendor problems when the contextual data contains intertemporal dependence and non-stationarities. While machine learning tools have observed increasing use in data-driven inventory management problems, most of the existing work assumes that the contextual data are independent and identically distributed (often referred to as i.i.d.). However, such assumptions are often violated in real operational environments where the contextual data are sequentially generated with intertemporal correlations and possible non-stationarities. By accommodating these naturally arising operational environments, our work adopts comparatively more realistic assumptions and develops out-of-sample performance bounds for learning data-driven contextual newsvendor problems.
Problem definition: We consider intertemporal pricing in the presence of reference effects and consumer heterogeneity. Our research question encompasses how to estimate heterogeneous consumer reference effects from data and how to efficiently compute the optimal pricing policy. Academic/practical relevance: Understanding reference effects is essential for designing pricing policies in modern retailing. Our work contributes to this area by incorporating consumer heterogeneity under arbitrary distributions. Methodology: We propose a mixed logit demand model that allows arbitrary joint distributions of valuations, responsiveness to prices, and responsiveness to reference prices among consumers. We use a nonparametric estimation method to learn consumer heterogeneity from transaction data. Further, we formulate the pricing optimization as an infinite horizon dynamic programming problem and solve it by applying a modified policy iteration algorithm. Results: Moreover, we investigate the structure of optimal pricing policies and prove the suboptimality of constant pricing policies even when all consumers are loss-averse according to the classical definition. Our numerical studies show that our estimation and optimization framework improves the expected revenue of retailers via accounting for heterogeneity. We validate our model using real data from JD.com, a large E-commerce retailer, and find empirical evidence of consumer heterogeneity. Managerial implications: In practice, ignoring consumer heterogeneity may lead to a significant loss of revenue. Furthermore, heterogeneous reference effect offers a strong motive for promotions and price fluctuations.
Problem definition: Shared micromobility vehicles provide an eco-friendly form of short-distance travel within an urban area. Because customers pick up and drop off vehicles in any service region at any time, such convenience often leads to a severe imbalance between vehicle supply and demand in different service regions. To overcome this, a micromobility operator can crowdsource individual riders with reward incentives in addition to engaging a third-party logistics provider (3PL) to relocate the vehicles. Methodology/results: We construct a time-space network with multiple service regions and formulate a two-stage stochastic mixed-integer program considering uncertain customer demands. In the first stage, the operator decides the initial vehicle allocation for the regions, whereas in the second stage, the operator determines subsequent vehicle relocation across the regions over an operational horizon. We develop an efficient solution approach that incorporates scenario-based and time-based decomposition techniques. Our approach outperforms a commercial solver in solution quality and computational time for solving large-scale problem instances based on real data. Managerial implications: The budgets for acquiring vehicles and for rider crowdsourcing significantly impact the vehicle initial allocation and subsequent relocation. Introducing rider crowdsourcing in addition to the 3PL can significantly increase profit, reduce demand loss, and improve the vehicle utilization rate of the system without affecting any existing commitment with the 3PL. The 3PL is more efficient for mass relocation than rider crowdsourcing, whereas the latter is more efficient in handling sporadic relocation needs. To serve a region, the 3PL often relocates vehicles in batches from faraway, low-demand regions around peak hours of a day, whereas rider crowdsourcing relocates a few vehicles each time from neighboring regions throughout the day. Furthermore, rider crowdsourcing relocates more vehicles under a unimodal customer arrival pattern than a bimodal pattern, whereas the reverse holds for the 3PL.
The coronavirus/SARS-CoV-2 (COVID-19) outbreak has caused severe supply chain disruptions in practically all industries worldwide. Online e-commerce platforms, which interact directly with various industries and service numerous consumers, have become remarkable interfaces to observe the impacts of the pandemic on supply chains. Using quantitative operational data obtained from JD.com https://www.jd.com., this study analyzes the impact of the pandemic on supply chain resilience, summarizes the challenging scenarios that retailing supply chains experienced in China, and presents the practical response of JD.com throughout the pandemic. To summarize, the pandemic caused exceptional demand and severe logistical disruptions in China, and JD.com has handled well its supply chain management in response based on its integrated supply chain structure and comprehensive intelligent platforms. In particular, the existing intelligent platforms and the delivery procedures were modified slightly but promptly to deal with specific disruptions. Moreover, the entire market scenario in China was effectively controlled through the joint efforts of multiple firms, the government, and the entire Chinese society. Our study provides an example of using practical operational indicators to analyze supply chain resilience, and suggests firms pay attention to operational flexibility and collaboration beyond supply chains to deal with a large-scale supply chain disruption, such as the COVID-19 outbreak.
We investigate a data-driven multiperiod inventory replenishment problem with uncertain demand and vendor lead time (VLT) with accessibility to a large quantity of historical data. Different from the traditional two-step predict-then-optimize (PTO) solution framework, we propose a one-step end-to-end (E2E) framework that uses deep learning models to output the suggested replenishment amount directly from input features without any intermediate step. The E2E model is trained to capture the behavior of the optimal dynamic programming solution under historical observations without any prior assumptions on the distributions of the demand and the VLT. By conducting a series of thorough numerical experiments using real data from one of the leading e-commerce companies, we demonstrate the advantages of the proposed E2E model over conventional PTO frameworks. We also conduct a field experiment with JD.com, and the results show that our new algorithm reduces holding cost, stockout cost, total inventory cost, and turnover rate substantially compared with JD’s current practice. For the supply chain management industry, our E2E model shortens the decision process and provides an automatic inventory management solution with the possibility to generalize and scale. The concept of E2E, which uses the input information directly for the ultimate goal, can also be useful in practice for other supply chain management circumstances.
New products are highly valued by manufacturers and retailers due to their vital role in revenue generation. Product life cycle (PLC) curves often vary by their shapes and are complicated by promotional activities that induce spiky and irregular behaviors. We collaborate with JD.com to develop a flexible PLC curve forecasting framework based on Bayesian functional regression that accounts for useful covariate information, including product attributes and promotion. The functional model treats PLC curves as target variables and includes both scalar and functional predictors, capturing time-varying promotional activities. Harnessing the power of basis function transformation, the developed model can effectively characterize the local features and temporal evolution of sales curves. Our Bayesian framework can generate initial curve forecasts before the product launch and update the forecasts dynamically as new sales data are collected. We validate the superior performance of our method through extensive numerical experiments using three real-world data sets. Our forecasting framework reduces the forecasting error by 5.35%–30.76% over JD.com's current model and outperforms alternative models significantly. Furthermore, the estimated promotion effect function provides useful insights into how promotional activities interact with sales curves.
New technologies and innovative business models are leading to connected, shared, autonomous, and electric solutions for the tomorrow of urban transport and logistics (UTL). The efficiency and sustainability of these solutions are greatly empowered by the capability of understanding and utilizing the tremendous amount of data generated by passengers, drivers, and vehicles. In this study, we first review the innovative applications in UTL and several related research areas in the operations management (OM)/operations research (OR) literature. We then highlight the sources, types, and uses of data in different applications. We further elaborate on business analytics techniques and software developed to facilitate the planning and management of UTL systems. Finally, we conclude the paper by reflecting on the emerging trends and potential research directions in data-driven decision making for smart UTL.
Problem definition: Observing the retail industry inevitably evolving into omnichannel, we study an offline-channel planning problem that helps an omnichannel retailer make store location and location-dependent assortment decisions in its offline channel to maximize profit across both online and offline channels, given that customers’ purchase decisions depend on not only their preferences across products but also, their valuation discrepancies across channels, as well as the hassle costs incurred. Academic/practical relevance: The proposed model and the solution approach extend the literature on retail-channel management, omnichannel assortment planning, and the broader field of smart retailing/cities. Methodology: We derive parameterized models to capture customers’ channel choice and product choice behaviors and customize a corresponding parameter estimation approach employing the expectation-maximization method. To solve the proposed optimization model, we develop a tractable mixed integer second-order conic programming reformulation and explore the structural properties of the reformulation to derive strengthening cuts in closed form. Results: We numerically validate the efficacy of the proposed solution approach and demonstrate the parameter estimation approach. We further draw managerial insights from the numerical studies using real data sets. Managerial implications: We verify that omnichannel retailers should provide location-dependent offline assortments. In addition, our benchmark studies reveal the necessity and significance of jointly determining offline store locations and assortments, as well as of incorporating the online channel while making offline-channel planning decisions.