Estimation of the covariance matrix of asset returns is crucial to portfolio construction. As suggested by economic theories, the correlation structure among assets differs between emerging markets and developed countries. It is therefore imperative to make rigorous statistical inference on correlation matrix equality between the two groups of countries. However, if the traditional vector-valued approach is undertaken, such inference is either infeasible due to limited number of countries comparing to the relatively abundant assets, or invalid due to the violations of temporal independence assumption. This highlights the necessity of treating the observations as matrix-valued rather than vector-valued. With matrix-valued observations, our problem of interest can be formulated as statistical inference on covariance structures under sub-Gaussian distributions, i.e., testing non-correlation and correlation equality, as well as the corresponding support estimations. We develop procedures that are asymptotically optimal under some regularity conditions. Simulation results demonstrate the computational and statistical advantages of our procedures over certain existing state-of-the-art methods for both normal and non-normal distributions. Application of our procedures to stock market data reveals interesting patterns and validates several economic propositions via rigorous statistical testing.
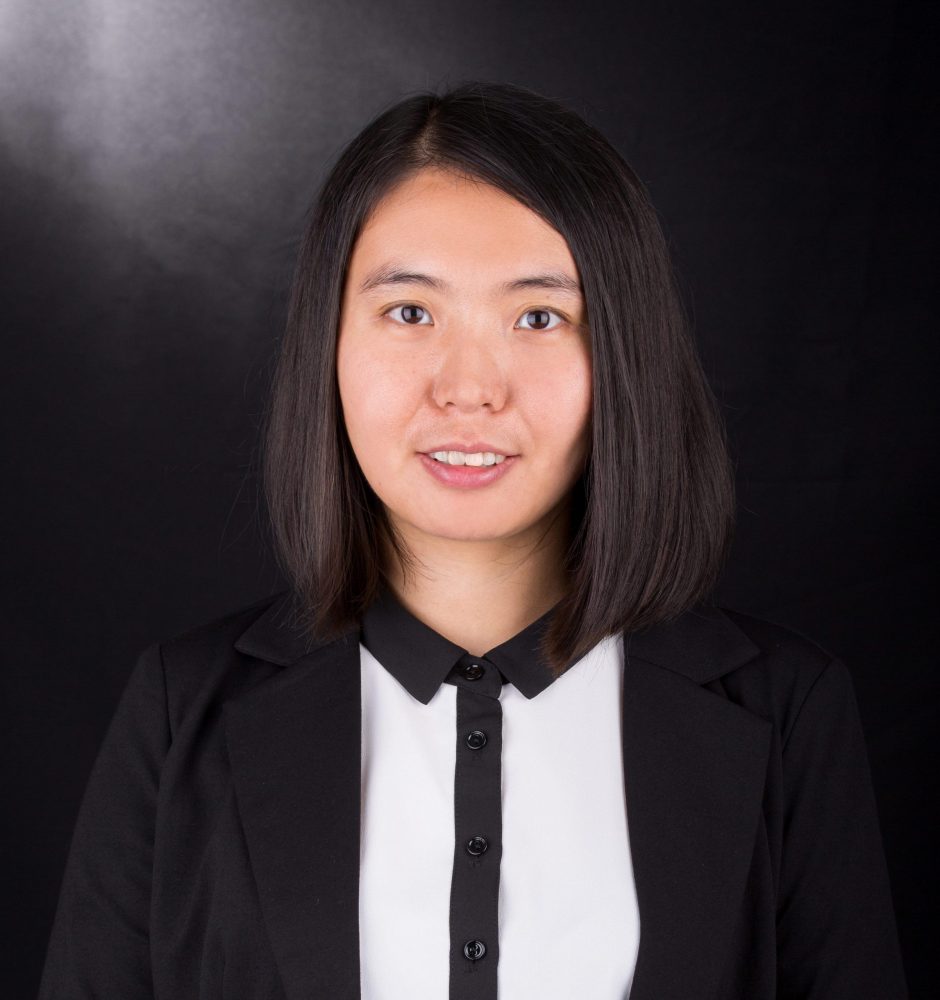
3917 0015
KK 816
- PhD in Statistics, The Wharton School of Business, University of Pennsylvania
- MS in Statistics, The Wharton School of Business, University of Pennsylvania
- BS in Statistics, School of Mathematical Sciences, Peking University
- BS in Economics, Center for Economic Research, Peking University
Dan Yang received her Ph.D. degree in Statistics from the Wharton School of Business, University of Pennsylvania in 2012. She is an assistant professor in the Department of Statistics and Biostatistics at Rutgers University from 2013.
- MSBA7011 Managing and Mining Big Data
- MSBA7013 Forecasting and Predictive Analytics
- High-dimensional statistical inference
- Dimension reduction
- Tensor data
- Big data
- Xin Chen, Dan Yang, Yan Xu, Yin Xia, Dong Wang, and Haipeng Shen (2023). Testing and Support Recovery of Correlation Structures for Matrix-Valued Observations with an Application to Stock Market Data. Journal of Econometrics, 232(2):544-564.
- Rong Chen, Dan Yang, and Cun-Hui Zhang (2022). Factor Models for High-Dimensional Tensor Time Series. Journal of the American Statistical Association, 117(537):94-116.
- Rong Chen, Han Xiao, and Dan Yang (2021). Autoregressive Models for Matrix-valued Time Series. Journal of Econometrics, 222(1):539-560.
- Dan Yang, Zongming Ma, and Andreas Buja (2016). Rate Optimal Denoising of Simultaneously Sparse and Low Rank Matrices. Journal of Machine Learning Research, 17:1-27.
- Gen Li, Dan Yang, Andrew B. Nobel, and Haipeng Shen (2016). Supervised Singular Value Decomposition and Its Asymptotic Properties. Journal of Multivariate Analysis, 146:7-17.
- Dan Yang, Zongming Ma, and Andreas Buja (2014). A Sparse Singular Value Decomposition Method for High-Dimensional Data. Journal of Computational and Graphical Statistics, 23(4):923-942.
- Dan Yang and Dylan S. Small (2013). An R Package and a Study of Methods for Computing Empirical Likelihood. Journal of Statistical Computation and Simulation, 83(7):1363-1372.
- Dan Yang, Dylan S. Small, Jeffrey H. Silber, and Paul R. Rosenbaum (2012). Optimal Matching with Minimal Deviation from Fine Balance in a Study of Obesity and Surgical Outcomes. Biometrics, 68(2):628-636.
NSF BIGDATA, Statistical Learning with Large Dynamic Tensor Data, 2017-2020
Large tensor (multi-dimensional array) data routinely appear nowadays in a wide range of applications, due to modern data collection capabilities. Often such observations are taken over time, forming tensor time series. In this paper we present a factor model approach to the analysis of high-dimensional dynamic tensor time series and multi-category dynamic transport networks. Two estimation procedures are presented along with their theoretical properties and simulation results. Two applications are used to illustrate the model and its interpretations.
In finance, economics and many other fields, observations in a matrix form are often generated over time. For example, a set of key economic indicators are regularly reported in different countries every quarter. The observations at each quarter neatly form a matrix and are observed over consecutive quarters. Dynamic transport networks with observations generated on the edges can be formed as a matrix observed over time. Although it is natural to turn the matrix observations into long vectors, then use the standard vector time series 2 models for analysis, it is often the case that the columns and rows of the matrix represent different types of structures that are closely interplayed. In this paper we follow the autoregression for modeling time series and propose a novel matrix autoregressive model in a bilinear form that maintains and utilizes the matrix structure to achieve a substantial dimensional reduction, as well as more interpretability. Probabilistic properties of the models are investigated. Estimation procedures with their theoretical properties are presented and demonstrated with simulated and real examples.