社交媒體盛行,不少人都會在平台上分享新聞,令新聞的傳播效果更加顯著,企業亦因此十分留心社交媒體上的新聞討論熱點,以便更貼近「民情」,亦慎防公關危機的發生。 港大經管學院市場學、創新及資訊管理學副教授潘光俊,早前就大眾閱讀新聞和分享社交媒體的行為發表研究報告,發現文章內容對大眾閱讀新聞和社交媒體分享的行為有影響。他指出,讀者傾向在新聞網站閱讀負面新聞,但在社交媒體上,卻傾向分享正面報道,「目的是為『推廣』自己,予人一個正面形象,並非側重所分享的新聞本身。」研究並發現,較深的文章亦傾向多人分享,但實際閱讀的人數卻很少。
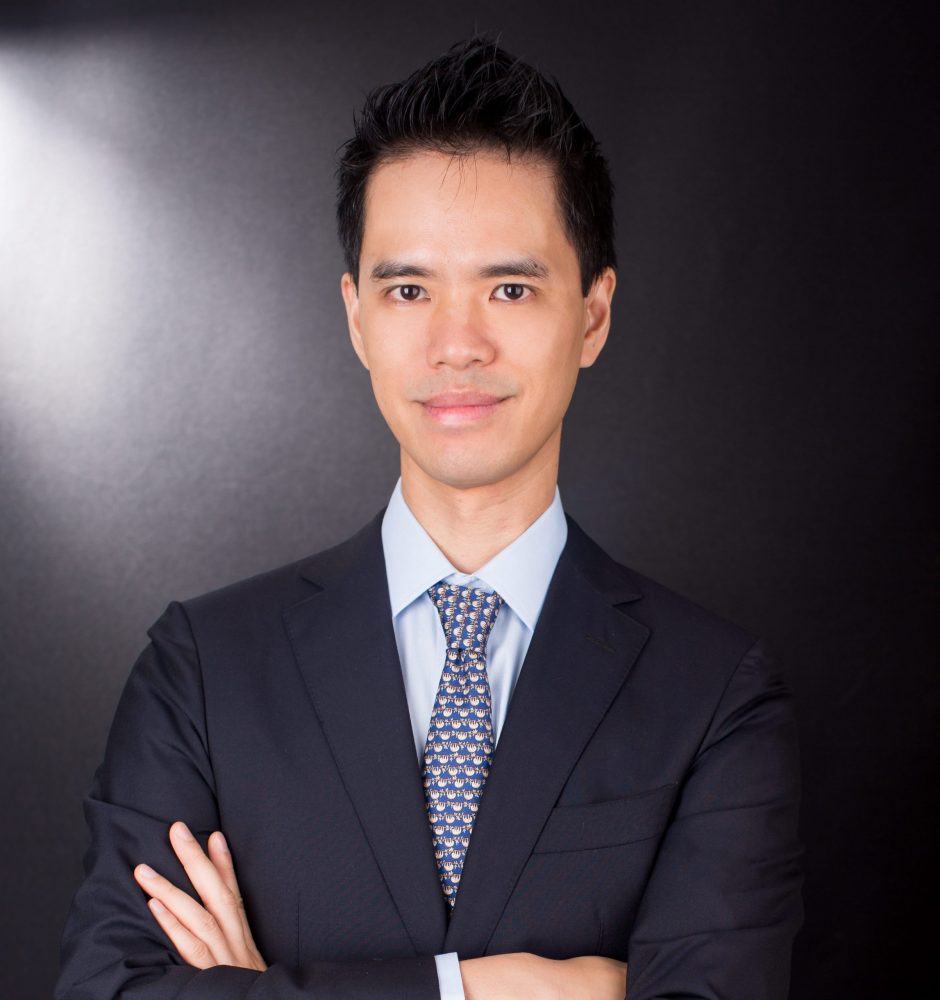
3917 4212
KK 704
As coronavirus rages across the globe, online business is still booming, with data and analytics driving this trend. People now marooned at home for the foreseeable future are finding the daily goods they need from online stores, solace in conferencing apps, and entertainment provided by streaming platforms. The world is revolving increasingly online with lockdowns in place, and data is being even further highlighted as an undisputable source of wealth.
潘博士是一位學者,商業顧問,企業家和標準舞舞者。作為人工智能和金融科技領域的專家,他最近加入了香港大學,並相信香港將會在中美的科技革命中的歷史時刻中扮演著關鍵的角色。
With the rapid growth of online social network sites (SNSs), it has become imperative for platform owners and online marketers to quantify what factors drive content production on these platforms. Previous research identified challenges in modeling these factors statistically using observational data, where the key difficulty is the inability of conventional methods to disentangle the effects of network formation and network influence on content generation from the subsequent feedback effect of newly generated content on network structure. In this paper, we adopt and enhance an actor-oriented continuous-time statistical model that enables the joint estimation of the coevolution of the users’ social network structure and of the amount of content they produce, using a Markov chain Monte Carlo–based simulation approach. Specifically, we offer a method to analyze nonstationary and continuous-time behavioral data, typically recorded in social media ecosystems, in the presence of network effects and other observable and unobservable user-specific covariates. The proposed method can help disentangle network effects of interest from feedback effects on the network. We apply our model to social network and public posting data over six months to find that (1) users tend to connect with others that have similar posting behavior; (2) however, after doing so, these users tend to diverge in their posting behavior, and (3) peer influence effects are sensitive to the strength of the posting behavior. More broadly, the proposed method provides researchers and practitioners with a statistically rigorous approach to analyze network effects in observational data. Our results lead to insights and recommendations for SNS platform owners on how to sustain an active and viable community.