雖然先進的機器學習算法大大提升了金融欺詐檢測研究的發展,然而,在構建有效辨識欺詐行為特徵(或屬性)方面仍然存在挑戰。近年,在人們可以於沒有金融中介的情況下向他人貸款的P2P 借貸平台上,出現了一種新型欺詐,因為信息不對稱的問題在這些借貸市場更加嚴重。我們以舞弊三角論及其引伸的理論為基礎,利用設計科學研究方法,除了參考借款人和貸款請求的基本特徵以外,更直接以P2P 借貸交易數據構建了五類新的行為特徵。這些行為特徵,以研究欺詐者的貸款請求和還款記錄、人際網絡、投標過程的特徵和活動次序為基礎,並旨在評估其進行欺詐的能力、誠信和欺詐機會。我們使用涵蓋中國兩個大型 P2P 借貸平台的真實用戶的數據集進行評估。研究結果顯示,結合新的行為特徵與基本特徵可顯著提升金融欺詐檢測的表現。是項設計科學研究為金融欺詐檢測的文獻和實踐帶來了新見解。
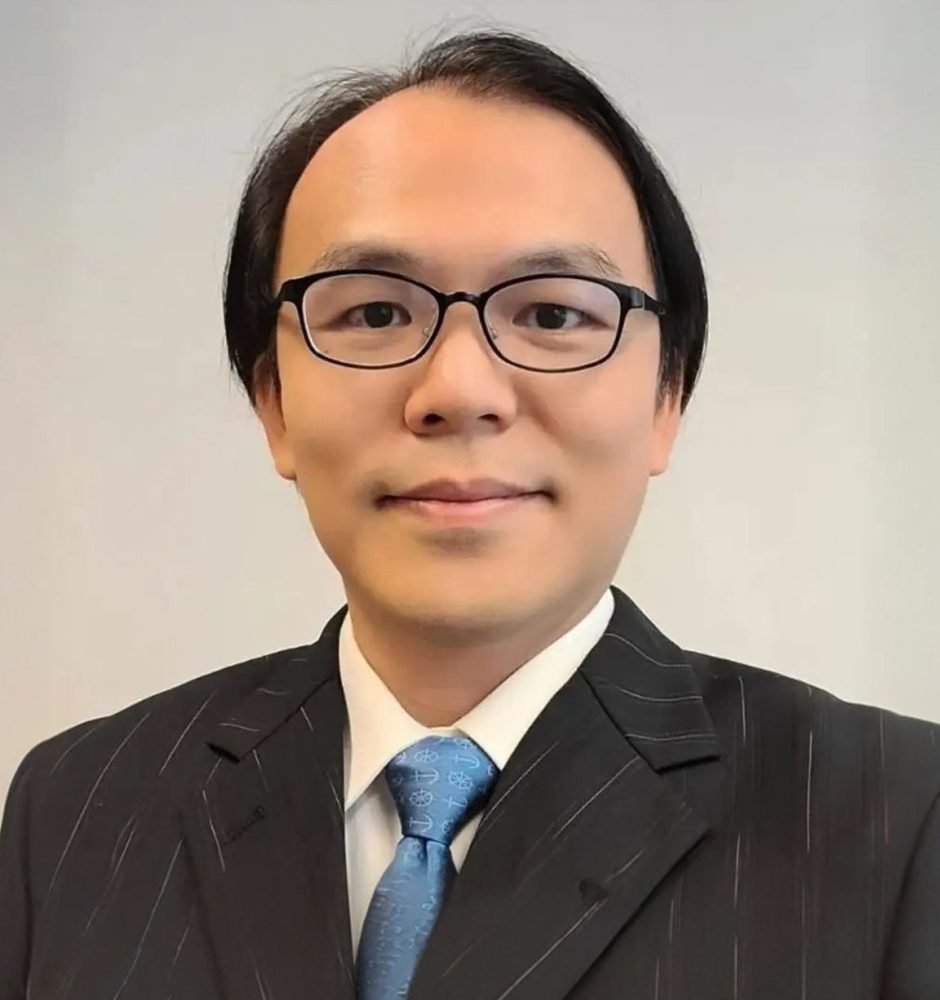
3917 1014
KK 1324
- Ph.D. (Management Information Systems), The University of Arizona
- B.Sc. (Computer Science (Information Systems)), The University of Hong Kong
Michael Chau is a Professor in Innovation and Information Management in the HKU Business School at the University of Hong Kong. He served as the Warden of Lee Chi Hung Hall (2009-2021) and the Program Director/Coordinator of the BBA (Information Systems) program (2006-2009, 2012-2018). He is also an Honorary Fellow of the HKU-HKJC Centre for Suicide Research and Prevention. He received a Ph.D. degree in Management Information Systems from the University of Arizona and a B.Sc. degree in Computer Science (Information Systems) from the University of Hong Kong. His research interests include business analytics, artificial intelligence, web mining and social media, electronic commerce, fintech, smart health, security informatics, human-computer interaction, and IT in education.
He has published more than 150 articles in premier journals and conferences in information systems, computer science, and information science. He has received multiple international research awards and has been highly ranked in several research productivity studies.
Michael has been active in serving the research community. He is a member of the AIS College of Senior Scholars and the Program Co-chair of PACIS 2024 and ICIS 2013. He has served on the organization committee and program committee of many information systems and computer science conferences, as well as the editorial board of multiple journals. He is a founding co-chair of the Pacific-Asia Workshop on Intelligence and Security Informatics (PAISI 2006-2019).
Michael has taught a wide range of courses at HKU at both the undergraduate and postgraduate levels, including database management, computer networking, business analytics and big data, artificial intelligence, project management, spreadsheet modelling, and computer programming.
- Business analytics and big data
- Artificial intelligence
- Web mining and social media
- Electronic commerce
- Fintech
- Smart health
- Security informatics
- Human-computer interaction
- IT in education
- Hu, J., Hu D., Yang X., and Chau, M. “The Impacts of Lockdown on Open Source Software Contributions During the COVID-19 Pandemic,” Research Policy, 52(10), 104885, 2023.
- Xu, J. J., Chen, D., Chau, M., Li, L., and Zheng, H. “Peer-to-Peer Loan Fraud Detection: Constructing Features from Transaction Data,” MIS Quarterly (MISQ), 46(3), pp. 1777-1792, 2022.
- Deng, B. and Chau, M. “The Effect of the Expressed Anger and Sadness on Online News Believability,” Journal of Management Information Systems (JMIS), 38(4), pp. 959-988, 2021.
- Chau, M., Li, W., Yang, B., Lee, A., and Bao, Z. “Incorporating the Time-Order Effect of Feedback in Online Auction Markets through a Bayesian Updating Model,” MIS Quarterly (MISQ), 45(2), pp. 985-1006, 2021.
- Chau, M., Li, T. M. H., Wong, P. W. C., Xu, J. J., Yip, P. S. F., and Chen, H. “Finding People with Emotional Distress in Online Social Media: A Design Combining Machine Learning and Rule-based Classification,” MIS Quarterly (MISQ), 44(2), pp. 933-955, 2020.
- Xu, J. J. and Chau, M. “Cheap Talk? The Impact of Lender-Borrower Communication on P2P Lending Outcomes,” Journal of Management Information Systems (JMIS), 35(1), pp. 53-85, 2018.
- Xu, J. J., Chau, M., and Tan, B. “The Development of Social Capital in the Collaboration Network of Information Systems Scholars,” Journal of the Association for Information Systems (JAIS), 15(12), pp. 835-859, 2014.
- Fang, X., Hu, P. J., Chau, M., Hu, H., Yang, Z., and Sheng, O. R. L. “A Data-Driven Approach to Measure Web Site Navigability,” Journal of Management Information Systems (JMIS), 29(2), pp. 173-212, 2012.
- Chau, M. and Xu, J. “Business Intelligence in Blogs: Understanding Consumer Interactions and Communities,” MIS Quarterly (MISQ), 36(4), pp. 1189-1216, 2012.
- Chau, M. “Visualizing Web Search Results Using Glyphs: Design and Evaluation of a Flower Metaphor,” ACM Transactions on Management Information Systems (ACM TMIS), 2(1), pp. 1-27, 2011.
- Cheng, R., Chau, M., Garofalakis, M., and Yu, J. X. “Mining Large Uncertain and Probabilistic Databases,” IEEE Transactions on Knowledge and Data Engineering (IEEE TKDE), 22(9), 1201-1202, 2010.
- Roussinov, D. and Chau, M. “Combining Information Seeking Services into a Meta Supply Chain of Facts,” Journal of the Association for Information Systems (JAIS), 9(3), 175-199, 2008.
- Xu, J., Wang, G., Li, J., and Chau, M. “Complex Problem Solving: Identity Matching Based on Social Contextual Information,” Journal of the Association for Information Systems (JAIS), 8(10), 525-545, 2007.
- Schroeder, J., Xu, J., Chen, H., and Chau, M. “Automated Criminal Link Analysis Based on Domain Knowledge,” Journal of the American Society for Information Science and Technology (JASIST), 58(6), 842-855, 2007.
- Chen, H., Chung, W., Xu. J., Wang, G., Qin, Y., and Chau, M. “Crime Data Mining: A General Framework and Some Examples,” IEEE Computer, 37(4), 50-56, 2004.
Dr. Chau’s research has appeared in more than 150 publications. Please refer to https://pweb.fbe.hku.hk/~mchau/publications.html for a complete list.
- INFORMS ISS Design Science Award (2020)
- IEEE ITSS Leadership Award in Intelligence and Security Informatics (2020)
- AIS Sandra Slaughter Service Award (2016)
- HKU Outstanding Young Researcher Award (2014)
- HKU Faculty Research Postgraduate Supervision Award (2020)
- HKU Faculty Knowledge Exchange Award (2013, 2016)
- Journal on Information Systems Education Best Paper Runner-up (2019)
- IEEE ISI Best Conference Paper Runner-up (2016)
- PACIS Best Conference Paper (2006)
- Keynote speaker/Invited speaker at more than 10 conferences and workshops
Major roles
- Warden, Lee Chi Hung Hall (2009-2021)
- BBA(IS) Program Director/Coordinator (2006-2009, 2012-2018)
- Program Co-chair, Pacific-Asia Conference on Information Systems (PACIS 2024)
- Program Co-chair, International Conference on Information Systems (ICIS 2013)
- Program Co-chair, IEEE International Conference on Intelligence and Security Informatics (ISI 2019)
- Founding Co-chair, Pacific-Asia Workshop on Intelligence and Security Informatics (PAISI 2006-2019)
情緒表達被網絡新聞廣泛地傳播。現時在研究讀者對網絡新聞的看法時,主要聚焦語境線索對讀者的推論和考量的影響;然而,憤怒、悲傷等分立情緒所扮演的角色卻被忽視。本文旨在填補相關研究領域的空隙,透過檢視網絡新聞所表達憤怒和悲傷的情緒,分析如何對讀者造成影響。利用情緒即社會信息(EASI)理論和評價傾向框架(ATF),我們發現網絡新聞表達的憤怒情緒會降低其可信度。但是,悲傷的情緒不會引起相同的效果。研究另一個測試進一步發現,表達憤怒的情緒,可以解釋為讀者對作者認知心力的看法:讀者認為,新聞標題表達憤怒的情緒,代表作者在撰寫新聞時缺乏認知心力,令新聞可信度降低。我們還展示了新聞可信度具有下游影響,可以影響各種社交媒體行為,包括閱讀、喜歡、評論和分享。本研究以網絡新聞上的分立情緒(即憤怒、悲傷)為焦點,拓展了認知評估和人際影響層面的知識。研究的結果具有實際意義,幫助社交媒體平台、新聞聚合平台和監管機構管理數字內容,防止假新聞傳播。
Online auction markets host a large number of transactions every day. The transaction data in auction markets are useful for understanding the buyers and sellers in the market. Previous research has shown that sellers with different levels of reputation, as shown by the ratings and comments left in feedback systems, enjoy different levels of price premiums for their transactions. Feedback scores and feedback texts have been shown to correlate with buyers’ level of trust in a seller and the price premium that buyers are willing to pay (Ba and Pavlou 2002; Pavlou and Dimoka 2006). However, existing models do not consider the time-order effect, which means that feedback posted more recently may be considered more important than feedback posted less recently. This paper addresses this shortcoming by (1) testing the existence of the time-order effect, and (2) proposing a Bayesian updating model to represent buyers’ perceived reputation considering the time-order effect and assessing how well it can explain the variation in buyers’ trust and price premiums. In order to validate the time-order effect and evaluate the proposed model, we conducted a user experiment and collected real-life transaction data from the eBay online auction market. Our results confirm the existence of the time-order effect and the proposed model explains the variation in price premiums better than the benchmark models. The contribution of this research is threefold. First, we verify the time-order effect in the feedback mechanism on price premiums in online markets. Second, we propose a model that provides better explanatory power for price premiums in online auction markets than existing models by incorporating the time-order effect. Third, we provide further evidence for trust building via textual feedback in online auction markets. The study advances the understanding of the feedback mechanism in online auction markets.
Many people face problems of emotional distress. Early detection of high-risk individuals is the key to prevent suicidal behavior. There is increasing evidence that the Internet and social media provide clues of people’s emotional distress. In particular, some people leave messages showing emotional distress or even suicide notes on the Internet. Identifying emotionally distressed people and examining their posts on the Internet are important steps for health and social work professionals to provide assistance, but the process is very time-consuming and ineffective if conducted manually using standard search engines. Following the design science approach, we present the design of a system called KAREN, which identifies individuals who blog about their emotional distress in the Chinese language, using a combination of machine learning classification and rule-based classification with rules obtained from experts. A controlled experiment and a user study were conducted to evaluate system performance in searching and analyzing blogs written by people who might be emotionally distressed. The results show that the proposed system achieved better classification performance than the benchmark methods and that professionals perceived the system to be more useful and effective for identifying bloggers with emotional distress than benchmark approaches.